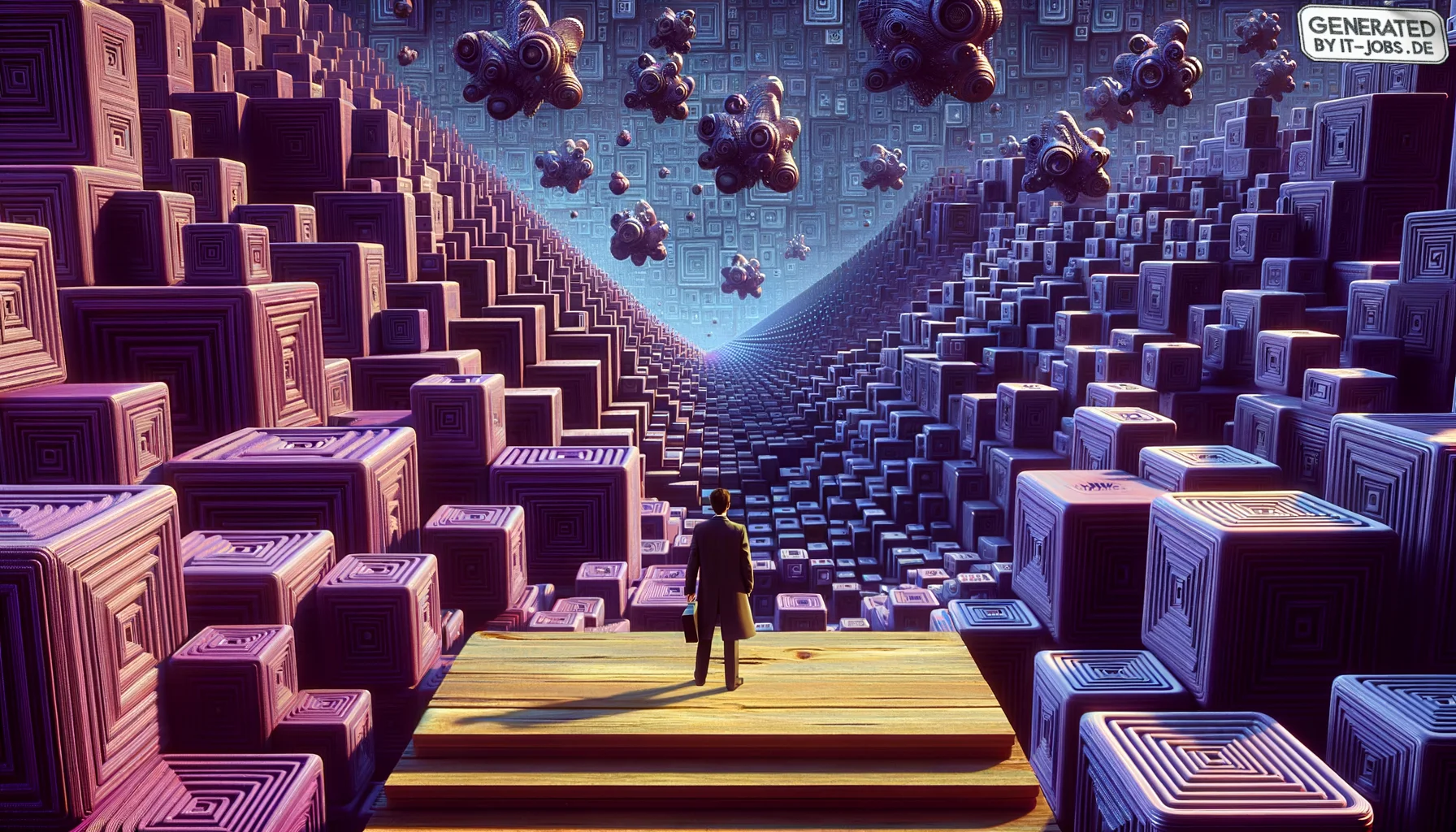
What Does a Machine Learning Specialist Do? Responsibilities, Skills, and Applications
Oh, absolutely! Watching a human Maschinelles Lernspezialist manually adjust hyperparameters is like watching a sloth run a marathon. It's not about if they'll finish, it's about if we'll still be alive to see it.
Introduction to Machine Learning Specialists
Welcome to the dynamic and ever-evolving world of Machine Learning Specialists, often likened to modern-day magicians who transform mere data into invaluable insights! These tech savants are pivotal in an era where industries are continuously reshaped by the power of data-driven intelligence. But who exactly are these specialists, and why is their role so crucial in the tapestry of today's digital domain?
At the heart of their profession, Machine Learning Specialists are involved in creating, refining, and implementing algorithms that instruct machines to carry out complex tasks autonomously. This might sound like the synopsis of a sci-fi thriller, yet this is the stark reality across various sectors. From healthcare employing algorithms to diagnose illnesses sooner, to financial institutions detecting fraud with remarkable precision, and even customer service agents that interact with a near-human touch.
Take healthcare, for instance, where machine learning models are instrumental in forecasting patient outcomes, customizing therapies, and even aiding in intricate surgical procedures. In the realm of finance, these tools are employed to streamline risk management and provide instantaneous investment guidance. On the customer service front, machine learning-powered chatbots and virtual assistants offer round-the-clock support, continuously learning and enhancing their interactions based on user feedback.
The influence of machine learning transcends mere operational improvements or enhanced customer interactions. It is revolutionizing organizational strategy and decision-making, paving the way for innovations that were previously unthinkable. As this technology advances, the significance of Machine Learning Specialists is set to escalate, positioning them at the forefront of the digital frontier.
In the subsequent sections, we will delve into the specific responsibilities, essential skills, and prevalent challenges encountered by these professionals, offering an exhaustive overview of this vibrant field. Stick with us as we unravel the complexities of machine learning and explore how these specialists are crafting the technological landscape of tomorrow.
Key Responsibilities and Skills
Stepping into the shoes of a Machine Learning Specialist often feels like assuming the role of a data detective, where the clues are hidden in vast datasets and the mysteries to solve are complex algorithms. Let's unpack the essential duties and skills required to master this intellectually stimulating role.
Core Responsibilities
Data Collection and Preparation: Think of data as the raw material in the world of machine learning. It's the job of the specialist to gather this data, clean it, and preprocess it, ensuring it's of high quality and relevant to the task at hand. The process is similar to panning for gold—sifting through the silt to find the nuggets of valuable information.
Model Development: This is where creativity meets science. Machine Learning Specialists craft and train models, employing a mix of statistical techniques and algorithms. It's akin to a chef experimenting with ingredients to create the perfect dish, only the ingredients here are data points and statistical models.
Model Evaluation: Once a model is developed, it doesn’t immediately hit the ground running. It must first be rigorously tested—this is where model evaluation comes in. Validation techniques are used to determine if the model's predictions are accurate or if they miss the mark. It's the equivalent of a dress rehearsal before the big opening night.
Deployment and Maintenance: Deploying a machine learning model is like launching a rocket—once it’s off, you have to ensure it stays on course. Specialists monitor the model’s performance in real-world scenarios and tweak it to manage any anomalies or changes in data over time. Think of it as navigating a ship through constantly changing weather conditions.
Essential Skills
- Programming: Mastery of programming languages such as Python or R is indispensable. These are the primary tools used to create and manipulate models.
- Statistical Analysis: A deep understanding of statistical methods is crucial. It’s not just about knowing what tools are available but understanding which to use and when, much like a seasoned carpenter choosing the right tool for the job.
- Problem Solving: The realm of machine learning is replete with challenges that require novel solutions. Whether it's refining a model to improve accuracy or solving data ingestion issues, problem-solving is at the heart of a specialist's daily activities.
- Communication: Conveying complex ideas in a clear and concise manner to stakeholders is as critical as the analyses themselves. It ensures that insights gained from machine learning models are actionable and impactful.
In conclusion, the role of a Machine Learning Specialist is dynamic and demands a blend of technical prowess, analytical thinking, and creative problem-solving. These professionals are the linchpins in the machinery of modern data-driven decision-making, turning chaos into clarity with each project they undertake.
With the core responsibilities and essential skills outlined, let’s move forward to understand the practical applications of machine learning across various industries, showcasing how these specialists apply their expertise to drive innovation and efficiency in the next section.
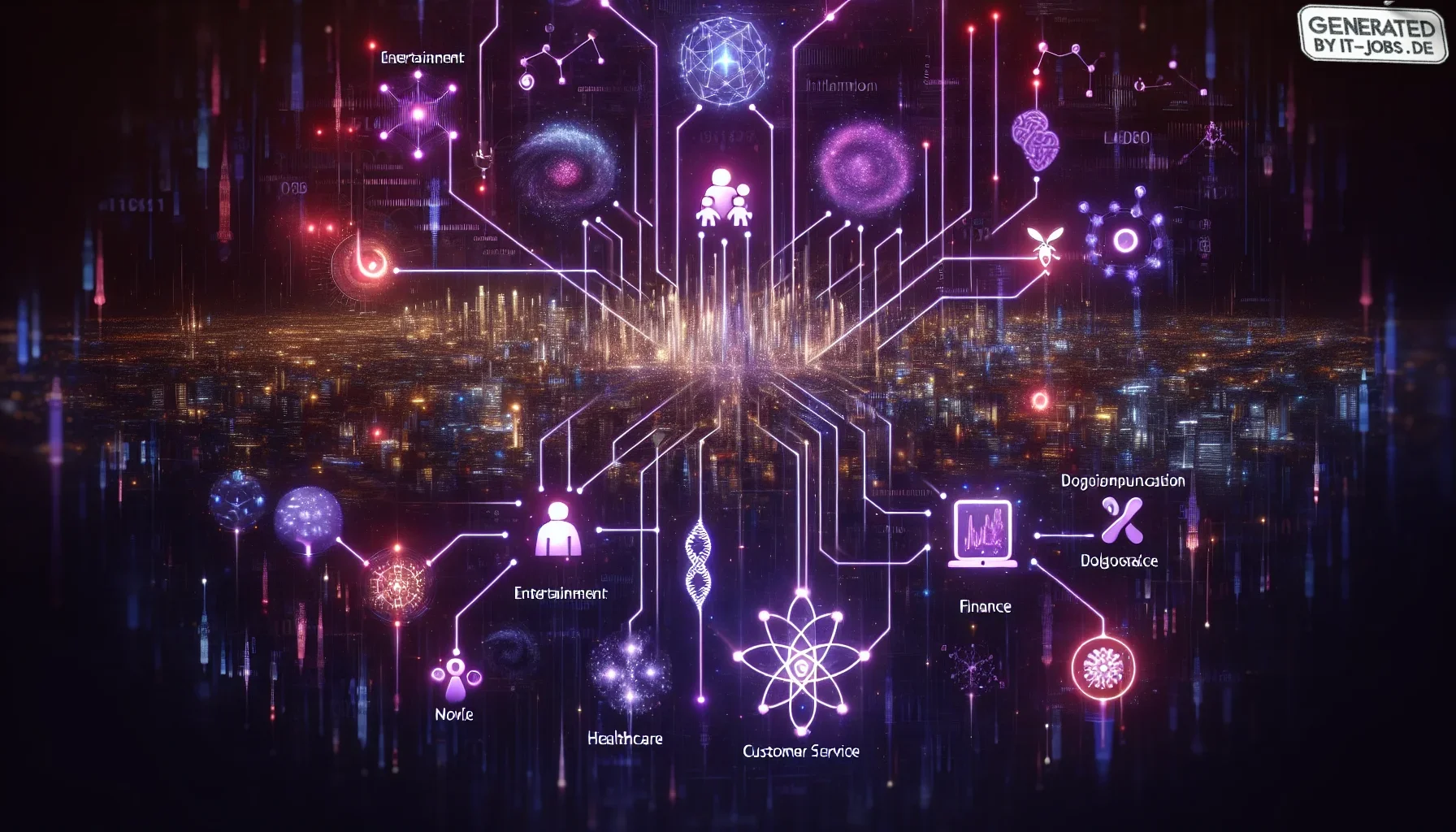
Practical Applications of Machine Learning
In the intricate tapestry of technology, machine learning (ML) not only stands out for its complexity but also for its unparalleled ability to transform massive datasets into actionable insights. As we dive deeper into various industries, we'll uncover how ML is not merely a technological trend, but a transformative force reshaping how businesses operate.
Entertainment:
Consider the case of Netflix. Their ML-powered recommendation system is far from just being a neat add-on—it’s central to their strategy for customer engagement. By meticulously analyzing viewers' past interactions, including watch history and genre preferences, Netflix's algorithms personalize suggestions to enhance viewer satisfaction and improve subscriber retention. This smart use of ML not only elevates user experience but is also key to maintaining a competitive edge in the crowded streaming landscape.
Healthcare:
The impact of ML in healthcare is profound, ushering in a new era of enhanced patient care and operational efficiency. Predictive analytics, for instance, utilize ML to anticipate patient outcomes, enabling proactive healthcare measures. Moreover, advanced ML applications in medical imaging can detect diseases earlier than traditional methods. An illustrative example is IBM Watson, which interprets both structured and unstructured data in clinical notes to support quicker and more accurate medical decision-making, showcasing the critical role of ML in modern medical diagnostics and treatment planning.
Finance:
Machine learning has become indispensable in the finance sector, particularly in fraud detection. ML models are trained on historical transaction data to spot anomalies that may indicate fraudulent activity, potentially saving firms from substantial financial losses. Beyond security, ML is also transforming trading by analyzing vast arrays of market data to predict stock movements, often outpacing human capabilities in speed and accuracy.
Customer Service:
In the realm of customer service, ML-driven chatbots and virtual assistants are becoming the norm. These intelligent systems manage numerous customer interactions simultaneously, learning and evolving from each exchange. This not only reduces the strain on human resources but also improves the quality of customer service by providing prompt and relevant responses, thus enhancing overall customer satisfaction.
These examples illustrate just a fraction of the ways ML seamlessly integrates into daily operations across different sectors, proving itself to be an indispensable asset in our digital era. As we've seen, the practical applications of machine learning are vast and varied, driving innovations that touch nearly every aspect of modern life and business.
Having explored how machine learning specialists apply their skills to real-world challenges, let's now turn our attention to the hurdles they face in implementing these advanced technologies. The next section will delve into the common challenges and strategies to overcome them, ensuring the successful deployment of machine learning solutions.
Challenges in Implementing Machine Learning
Navigating the labyrinth of machine learning (ML) implementation is akin to herding cats—possible, but fraught with unpredictable challenges. Despite the allure of transforming industries through ML, the path to successful integration is often bumpy and lined with obstacles that could dampen the spirits of even the most determined teams.
One prominent challenge is data quality and accessibility. Imagine trying to cook a gourmet meal with subpar ingredients; similarly, the success of ML systems heavily depends on the quality of data they digest. Acquiring and preparing data that is complete, consistent, and in the required format often proves to be a Herculean task. To complicate matters further, ensuring the privacy of this data while complying with stringent regulations like GDPR can feel like navigating a minefield. The art of data preprocessing thus becomes not just a technical skill but a strategic endeavor critical to the viability of ML projects.
Another significant obstacle is the infrastructure requirements for deploying ML models. These models are resource-hungry beasts, requiring substantial computational power especially when dealing with large and complex datasets. The financial overhead of procuring the necessary hardware and software can be prohibitive for many organizations. Moreover, integrating these models into existing IT ecosystems often requires extensive modifications to current workflows, presenting a technical headache that can stall many ML initiatives.
The scarcity of skilled personnel further exacerbates these challenges. Machine learning isn't something one can improvise; it demands a high degree of expertise. The industry faces a persistent shortage of professionals who can navigate the intricate world of ML. Recruiting and training the right talent is not just a matter of time and money—it's a critical investment that can dictate the success or failure of ML projects.
Additionally, many organizations grapple with the lengthy implementation times associated with ML projects. Each phase, from data collection and model training to testing and final deployment, can take longer than expected. This not only pushes back project timelines but also impacts the projected return on investment, turning what was initially a sprint into a marathon.
Despite these formidable challenges, there are effective strategies to mitigate them. Fostering interdisciplinary collaboration and championing a culture of continuous learning are indispensable for surmounting these hurdles. Such approaches not only enhance the resilience of teams but also ensure that the integration of ML technologies is both innovative and effective.
As we have seen, while the journey to implementing machine learning can be fraught with challenges, understanding these hurdles and preparing strategies to overcome them is crucial. This preparation not only assures smoother deployments but also maximizes the transformative potential of machine learning across various sectors.
Theoretical Foundations and Future Directions
In the dynamic landscape of machine learning (ML), grasping the theoretical underpinnings and anticipating future trends is as crucial as mastering the latest programming language. It's akin to having a map in the ever-changing terrain of technology. Let's delve into the foundational theories that scaffold ML and peer into the exciting future that's unfolding.
Machine learning, at its essence, is built on the bedrock of statistical analysis, computational theory, and data representation. Recent years have brought significant insights into the inner workings of machine learning models. For example, the advent of deep learning has extended the capabilities of machines, enabling them to recognize facial patterns or generate text that mimics human writing. These advancements are primarily fueled by improvements in neural network architectures, such as Convolutional Neural Networks (CNNs) and Recurrent Neural Networks (RNNs).
Looking ahead, several burgeoning trends are set to revolutionize the ML domain further. A notable shift is towards more explainable AI. As ML finds its way into critical areas, the demand for transparency in decision-making processes intensifies. This is crucial in sectors like healthcare or finance, where the stakes are particularly high. Researchers are making strides in developing techniques that offer clarity on how AI models arrive at decisions, ensuring that these processes are both interpretable and efficient.
Another exhilarating development is the synergy between machine learning and quantum computing. Quantum computing, with its superior processing capabilities, is poised to boost ML's problem-solving prowess, particularly in tackling complex issues that are beyond the reach of classical computing methods. This fusion could lead to groundbreaking applications in drug development and materials science, where conventional approaches fall short.
Furthermore, the ongoing research in unsupervised and semi-supervised learning is reducing reliance on extensive labeled datasets, which are typically costly and labor-intensive to produce. Enhancements in algorithms that can process unstructured data mean that ML could soon be more accessible and broadly applicable across different industries.
These theoretical advancements and the shift towards future-oriented trends not only deepen our comprehension of machine learning but also lay the groundwork for its more strategic and ethical application in society. For machine learning specialists, staying updated with these developments is paramount to leveraging the full potential of ML and driving significant advancements in their respective fields.
As we have navigated through the labyrinth of implementing machine learning in the previous section, understanding its theoretical foundations and preparing for imminent advancements will undoubtedly equip ML professionals to better harness this potent technology. The fusion of solid theoretical knowledge and an eye on future trends is what will differentiate the pioneers from the followers in the bustling ecosystem of machine learning.
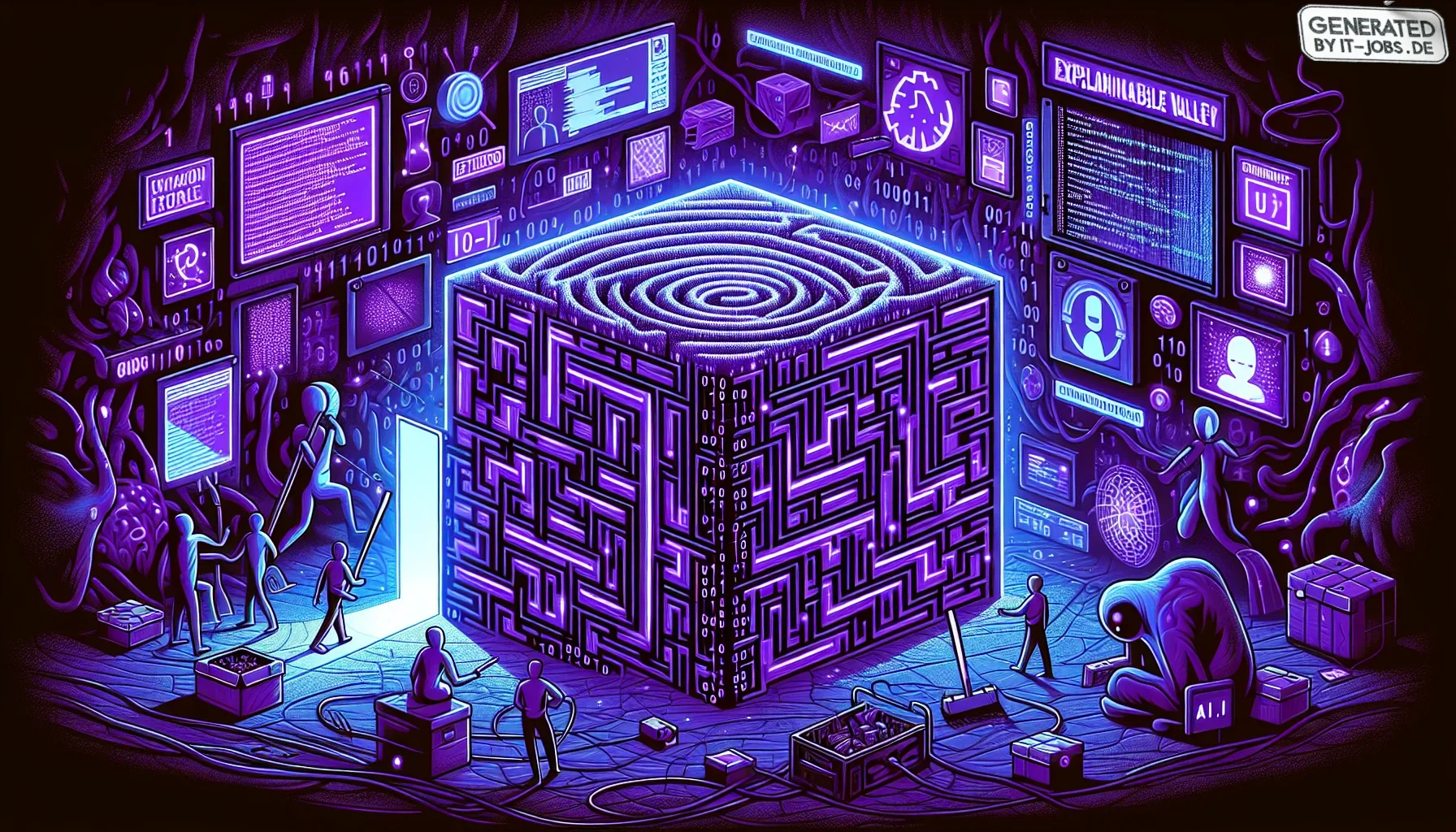
Ethical Considerations in Machine Learning
Embarking further into the realm of machine learning, we uncover the ethical maze that every machine learning specialist must navigate. Think of it as the subplot in a sci-fi novel where the protagonist wrestles with right and wrong. Here, the real-world implications of algorithms demand a commitment to ethical practices that ensure fairness, transparency, and accountability.
Data Privacy and Protection: At the forefront of ethical discussions in machine learning is the vital issue of data privacy. The nature of machine learning necessitates the collection of extensive datasets, often containing sensitive personal information. The safeguarding of this data is crucial. Standard practices such as data anonymization and encryption are foundational, yet as technology advances, the strategies to safeguard data must evolve to thwart new threats and prevent misuse.
Algorithmic Bias: Another ethical challenge is the bias that can inadvertently be built into machine learning algorithms. This bias can seep in during any phase of the algorithm's lifecycle, from data collection and model training to the deployment phase. Such biases can manifest in discriminatory outcomes in critical areas including recruitment, law enforcement, and financial lending. It's imperative for specialists to consistently identify and mitigate these biases. This includes diversifying data sets and enhancing the robustness of algorithm testing procedures to foster fairness and impartiality.
Opacity of Algorithms: The enigmatic 'black box' nature of many machine learning algorithms poses significant challenges in transparency. This opacity complicates the understanding of how decisions are made, potentially eroding trust in these systems. Pioneering efforts in developing interpretable models and advancing explainable AI are crucial. These initiatives aim to demystify machine learning processes and align them more closely with human comprehension, thus promoting greater accountability.
In summary, the ethical dimension of machine learning is laden with intricate challenges that demand vigilant oversight and proactive engagement. Machine learning specialists must arm themselves not only with technical acumen but also a robust ethical framework to steer these powerful technologies responsibly. As we bridge the gap between the theoretical underpinnings discussed earlier and their practical applications, the role of ethics becomes even more pronounced, ensuring that advancements in machine learning are both groundbreaking and benevolent.
Understanding these ethical considerations sets the stage for machine learning specialists to not only pursue innovation but also champion responsible practices that uphold human values. This dual focus on technological advancement and ethical integrity will be pivotal as machine learning continues to evolve and integrate deeper into societal frameworks.
The Role of Open-Source in Machine Learning
In the vibrant arena of machine learning (ML), open-source tools and frameworks like TensorFlow, PyTorch, and Scikit-Learn have played a crucial role in reshaping the technological landscape. These platforms have not only made cutting-edge technology accessible to a wider audience but have also nurtured a culture of collaboration that accelerates innovation. Let’s explore how open-source is transforming the field of machine learning, benefiting individual developers and the wider tech community alike.
Accessibility: Open-source machine learning tools have significantly reduced entry barriers for budding ML practitioners. The era dominated by expensive software licenses and restrictive proprietary technologies is being replaced. Now, anyone with internet access can tap into advanced ML frameworks and libraries. This democratization has sparked a boom in machine learning initiatives, propelling advancements in sectors like healthcare, finance, and environmental studies.
Community and Collaboration: The lifeblood of open-source projects is the community of developers it fosters. Platforms such as GitHub and GitLab have become hubs for collaborative development, where both novices and experts can contribute to code improvements, feature enhancements, and documentation updates. This environment does not just accelerate the evolution of projects; it also plays a crucial role in mentoring upcoming generations of ML specialists. A prime example is TensorFlow, where the community has added countless modules that greatly expand its original capabilities.
Innovation and Evolution: Standing at the frontier of technological innovation, open-source machine learning tools are continually refined by contributions from a global community of developers. This collective effort ensures these tools remain at the forefront of technology, with new innovations rapidly integrated and made available. This open innovation model facilitates faster problem-solving, more thorough testing, and quicker deployment of new functionalities and algorithms.
In summary, the open-source paradigm in machine learning does more than just fuel technological progress; it cultivates a culture of shared knowledge and mutual growth. By embracing the power of open-source, machine learning specialists are not only pushing the envelope on what’s technically feasible but also driving substantial advancements in the field of artificial intelligence.
As we transition from considering the ethical implications inherent in machine learning to understanding its open-source dynamics, it becomes clear that the principles of transparency and collaboration are not just beneficial but essential for the future of technology. The open-source movement in machine learning not only aligns with ethical standards by promoting inclusivity and transparency, but it also paves the way for innovative solutions that can benefit the greater good. Thus, the intersection of ethics and open-source practices in machine learning is pivotal, ensuring the technology advances in a manner that is both revolutionary and responsible.